UPCARS EN
- Affordable SaaS for SME
- Context aware
- Ensuring user privacy
- Quick set-up
Features
CONTEXT AWARE
Recommend items to users
Context awareness increases the relevance of the recommendation by adapting them to the situation of the user: do you need a sun creme in December if you are not living in the south hemisphere?
UPCARS deduces the context from the data and combined pre-filled and context modeling to deliver context aware recommendations. .
Delivering personalized recommendations to users help businesses to sell more, to increase their profitability and to gain customer loyalty.
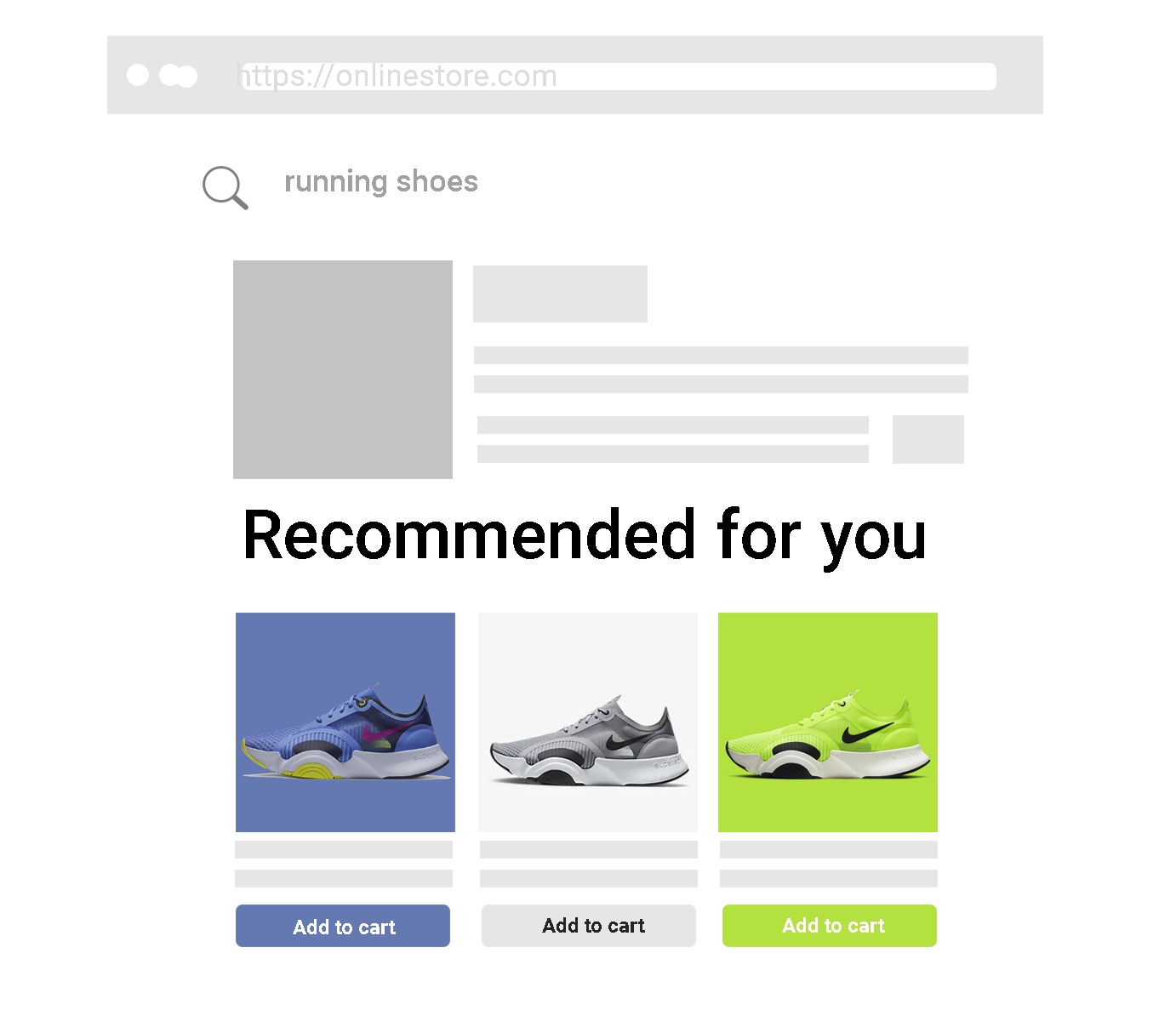
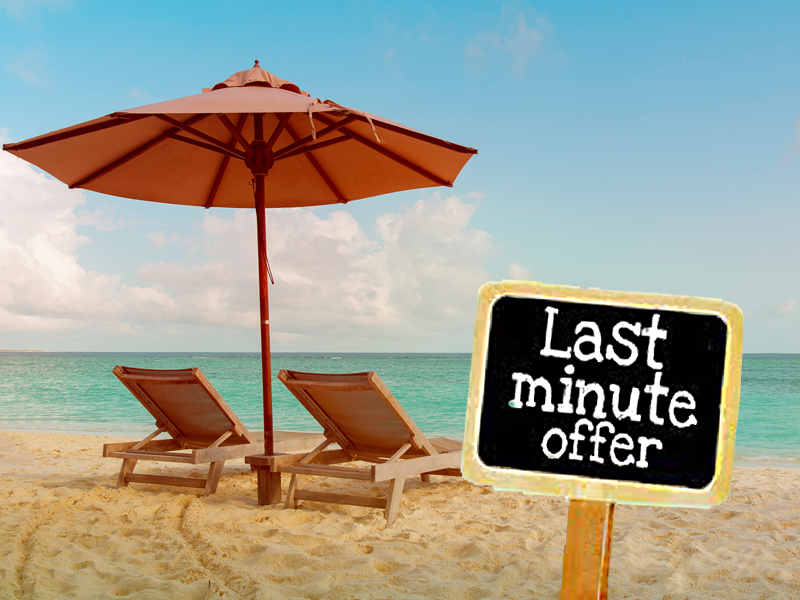
USER PRIVACY
Recommend users to items
UPCARS doesn't rely on user profiling that most of the RS content based are using at the expense of user privacy.
UPCARS algorithms only leverage the history of interactions between users and items, for exemple ratings, purchases, searches...
UPCARS keeps users anonymous and features less (no name, no gender, no age... are required).
Recommending users to items help businesses to sell unsold items (stocks, free seats...) through exclusive offers or las t minute deals.
NATURAL LANGUAGE SEARCH
Personalized search
Searching and recommending are the both side of the same coin.
Combining search and recommendation lead to more relevant and highly personalized recommendations.
User experience is enhanced by searches made in natural language, which results easier and faster than the traditional filtering and sorting approach.
UPCARS smart search is powered by Elasticsearch to store, browse and analyze large data volumes in real-time.
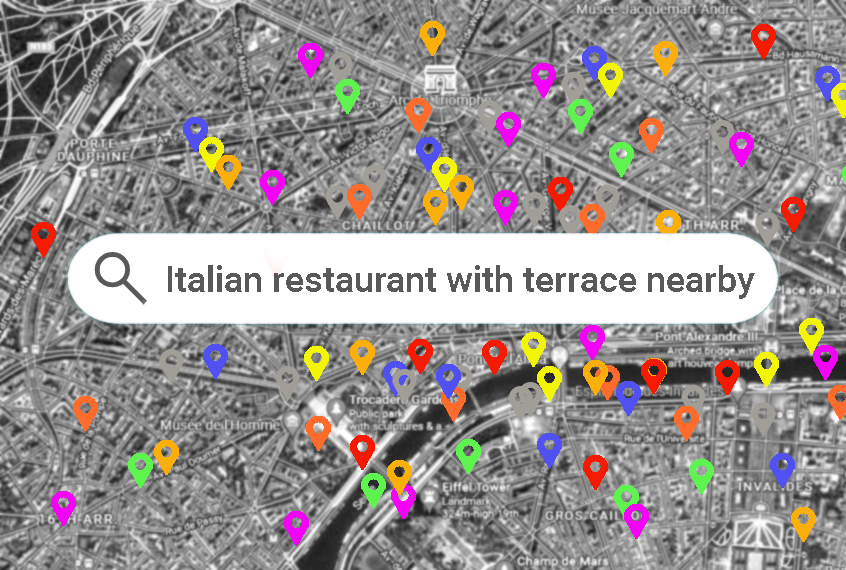
Check how UPCARS improves your business
HoReCa
Fill in available capacity (restaurant, hotels, trips) with lazer thin targeted last minute offers.
Market place
Increase partners and customers loyalties with relevant item recommendations to user and relevant user recommendations to items.
Retail
Stand out from competition, switch from competing on price to offer hyper personalization.
Digital publishers
Keep users engaged with your content. Deliver a great user experience.
Networking
Recommend business or personal connections. Find more accurate and faster matching between users.
Our technology
We developped a unique set of algorithms to fit with a wide range of data profiles and to deliver according to your business goals.
Human expertise combined with a proprietary algorithm enable NEXiT to quickly select the best RS for your use case.
KNN
K-Nearest Neighbor is a popular approach in collaborative filtering because of the intuitive interpretability. To estimate the rating that a user u would give to an item i, from all the users who have rated the item i, the most similar k users to u (called neighbors of u) are selected and the average of their rating is the estimated rating that u would give to i.
KNN is very accurate in estimating ratings, however it has disadvantages in terms of coverage and serendipity, so it would not give surprise recommendations as often as other algorithms.
Rectricted KNN
Restricted K-Nearest Neighbor is a version of the KNN that uses the same neighbours (global neighbours) to estimate the rating of all items. Note that these ratings cannot be estimated for items that have not being rated by any of the global neighbors.
Restricted KNN gives even safer recommendations than the usual KNN but is lacking serendipity providing conservative recommendations..
Bayesian times SVD++
Bayesian times Singular Value Decomposition ++ is an interesting approach, because it implicitly takes into account the context as the timeline of the review, but it proved to be difficult to properly tune. It is based on Bayesian matrix factorization, which is a probabilistic version of the SVD.
It is one of the state-of-the-art models and performs well on large datasets, however, it comes with a higher cost of training and tuning.
SVD
Singular Value Decomposition is the most popular matrix-factorization method that is used to reducing the number of features. Using this approach to recommender systems gained popularity in the context of the Netflix Prize, because of the advantage of latent features. These are hidden items features that cannot be identified by humans, but make sense for the algorithm. To adapt the linear algebra method to the recommender systems framework, the algorithm also considers the bias in ratings of different users.
In terms of advantages and cost, it is one of the most well-balanced approaches towards collaborative filtering recommender systems.
RGSVD
Riemannian Geometry SVD is a novel approach introduced by NEXiT, it uses unpublished research that cannot be detailed at this moment.
It is a high precision algorithm, context aware, versatile and scalable. It is highly serendipitous without reducing the accuracy.
RS edge technology
There is no onse-size-fits-all RS algorithm, we continuously develop new approaches to improve RS performance and our current research focuses on several directions such as knowledge graph, differential geometry and topology applications to RS.
Our research team is workingdaily to improve performance and to wider the applicable use cases of our RS .
NLP
Our natural language search engine uses the same advanced algorithms as the famous GPT-3 to extract tags as product names, category names, etc, from a natural language request and translate it into machine readable query processed by the Smart Search.
NLP provides great customer experience, however it requires to be trained to the business domain.
Smart Search
We have built a versatile search engine based on Elasticsearch. Boosters can be applied to push forward some products or features in the search ranking and maximize business goals.
Combining smart search with recommendations delivers more relevant recommendations
Why to choose UPCARS
QUICK SET-UP
UPCARS embeds tuning and admin tools to shorten time to set-up. Your RS will be up and running in just few days (from 2 to 20 days).
VERSATILE,SCALABLE
UPCARS leverage several RS algorithms to cope with a wider reange of use cases adn business goals. Our server are hosted in Amazon Cloud to ensure reliability and scalability.
EXPERT SUPPORT
Our team will partner with your experts to select the most appropriate RS for your use case and business goals. Or will handle the full process on your behalf.
CONTEXT AWARE
Recommendations context aware are much more relevant to users. UPCARS automatically analyzes the customer data set to extract the relevant context for your use case.
USER PRIVACY
Users are more and more concerned by their privacy and will be more and more reluctant to provide personal data against ‘free’ services. UPCARS mimnimize user data and ensure full privacy.s
SMART SEARCH
Smart search is a powerful ally of recommender systems, helping to provide more relevant results even when RS reaches its limit.
Feel free to contact us. We will come back to you asap.
Write us at contact@nextitproject.ro